外部のLLMをインストールする際、ollama.createを実行すると、「ResponseError:invalid digest format」というエラーが発生する場合があります。
本記事では、このエラーの原因と解消方法について分かりやすく解説します。
ollama.createのエラー「ResponseError:invalid digest format」
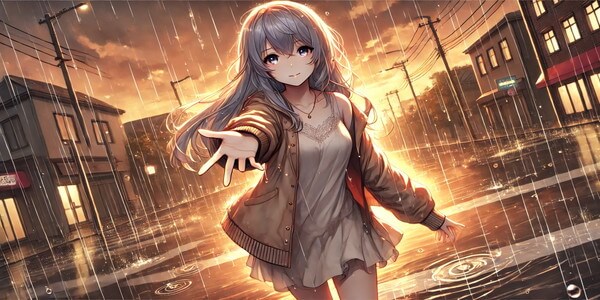
ollamaでは、外部からインストールしたLLMのモデルを利用することができます。
ollama.createを使用してモデルをセットアップする際に、「ResponseError: invalid digest format」というエラーが発生する場合があります。
このエラーの原因と解消する方法を詳しく解説します。
Ollamaの環境構築
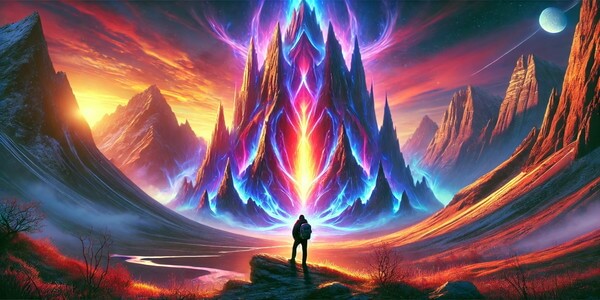
Dockerで環境構築
Dockerを使用してOllamaの環境構築をします
Dockerの使い方は以下の記事をご覧ください。
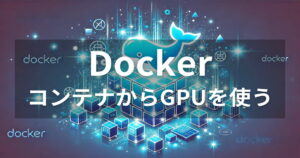
Ubuntuのコマンドラインで、Dockerfileを作成します。
mkdir ollama
cd ollama
nano Dockerfile
Dockerfileに以下の記述を貼り付けます。
# ベースイメージ(CUDA)の指定
FROM nvidia/cuda:12.1.0-cudnn8-devel-ubuntu22.04
# 必要なパッケージをインストール
RUN apt-get update && apt-get install -y python3-pip python3-venv git nano curl
RUN curl -fsSL https://ollama.com/install.sh | sh
# 作業ディレクトリを設定
WORKDIR /app
# アプリケーションコードをコピー
COPY . /app
# Python仮想環境の作成
RUN python3 -m venv /app/.venv
# 仮想環境をアクティベートするコマンドを.bashrcに追加
RUN echo "source /app/.venv/bin/activate" >> /root/.bashrc
# JupyterLabのインストール
RUN /app/.venv/bin/pip install Jupyter jupyterlab
# ollamaのインストール
RUN /app/.venv/bin/pip install ollama
# コンテナの起動時にbashを実行
CMD ["/bin/bash"]
FROM nvidia/cuda:12.1.0-cudnn8-devel-ubuntu22.04
CUDA12.1のベースイメージを指定しています。
RUN apt-get update && apt-get install -y python3-pip python3-venv git nano curl
必要なパッケージをインストールしています。
RUN curl -fsSL https://ollama.com/install.sh | sh
Linux版のOllamaをインストールしています。PythonでOllamaを動かす際にもLinux版Ollamaのインストールが必要になりますのでご注意ください。
RUN /app/.venv/bin/pip install Jupyter jupyterlab
JupyterLabをインストールしています。
RUN /app/.venv/bin/pip install ollama
Ollamaのパッケージをインストールしています。
LLMはOllamaのライブラリを使って動かしますので、PyTorchやTransformerは別途インストール不要です。
docker-compose.ymlでDockerコンテナの設定をします。
docker-compose.ymlのYAMLファイルを作成して開きます。
nano docker-compose.yml
以下のコードをコピーして、YAMLファイルに貼り付けます。
services:
langchain_rag:
build:
context: .
dockerfile: Dockerfile
image: langchain_rag
runtime: nvidia
container_name: langchain_rag
ports:
- "8888:8888"
volumes:
- .:/app/langchain_rag
deploy:
resources:
reservations:
devices:
- driver: nvidia
count: 1
capabilities: [gpu]
command: >
bash -c '/usr/local/bin/ollama serve & /app/.venv/bin/jupyter lab --ip="*" --port=8888 --NotebookApp.token="" --NotebookApp.password="" --no-browser --allow-root'
bash -c ‘/usr/local/bin/ollama serve & /app/.venv/bin/jupyter lab –ip=”*” –port=8888 –NotebookApp.token=”” –NotebookApp.password=”” –no-browser –allow-root’
bash -c '/usr/local/bin/ollama serve
Ollama Serverを起動しています。PythonのOllamaを使用する際に、Ollama Serverを起動しておく必要がありますので、ご注意ください。
& /app/.venv/bin/jupyter lab --ip="*" --port=8888 --NotebookApp.token="" --NotebookApp.password="" --no-browser --allow-root'
JupyterLabを8888番ポートで起動しています。
Dockerfileからビルドしてコンテナを起動します。
docker compose up
Dockerの起動後にブラウザの検索窓に”localhost:8888″を入力すると、Jupyter Labをブラウザで表示できます。
localhost:8888
エラーの確認と解消方法
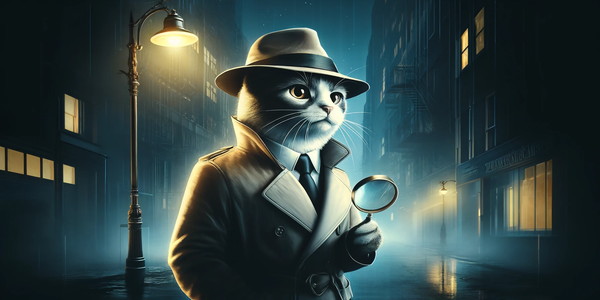
Dockerコンテナで起動したJupyter Lab上でOllamaの実装をします。
コードの実行中のログを記録するloggingモジュールの設定をします。
import logging
logging.basicConfig(level=logging.DEBUG)
日本語LLMモデル「tokyotech-llm-Llama-3.1-Swallow-8B-Instruct-v0.1-Q4_K_M.gguf」をダウンロードします。
!curl -L -o tokyotech-llm-Llama-3.1-Swallow-8B-Instruct-v0.1-Q4_K_M.gguf "https://huggingface.co/mmnga/tokyotech-llm-Llama-3.1-Swallow-8B-Instruct-v0.1-gguf/resolve/main/tokyotech-llm-Llama-3.1-Swallow-8B-Instruct-v0.1-Q4_K_M.gguf?download=true"
Llama 3.1 Swallowについては、別の記事で詳しく解説しています。
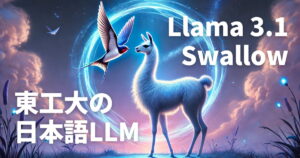
LLMの実行にはOllamaを使用します。
LLMのモデルがOllama使えるようにプロンプトテンプレートを指定して、モデルを作成します。
import ollama
modelfile='''
FROM ./tokyotech-llm-Llama-3.1-Swallow-8B-Instruct-v0.1-Q4_K_M.gguf
TEMPLATE """{{ if .System }}<|start_header_id|>system<|end_header_id|>
{{ .System }}<|eot_id|>{{ end }}{{ if .Prompt }}<|start_header_id|>user<|end_header_id|>
{{ .Prompt }}<|eot_id|>{{ end }}<|start_header_id|>assistant<|end_header_id|>
{{ .Response }}<|eot_id|>"""
PARAMETER stop "<|start_header_id|>"
PARAMETER stop "<|end_header_id|>"
PARAMETER stop "<|eot_id|>"
PARAMETER stop "<|reserved_special_token"
'''
ollama.create(model='swallow3_1', modelfile=modelfile)
FROM ./tokyotech-llm-Llama-3.1-Swallow-8B-Instruct-v0.1-Q4_K_M.gguf
ダウンロードしたモデルのパスが入ります。
TEMPLATE “””{{ if .System }}<|start_header_id|>system<|end_header_id|>…
モデルで使用するプロンプトテンプレートが入ります。
ollama.create(model=’swallow3_1’, modelfile=modelfile)
モデルとプロンプトテンプレートを使ってOllama用のモデルを作成します。model
にはOllamaで呼び出す際に使用する名前をつけられます。
Ollamaの詳しい使い方は、別の記事で解説しています。
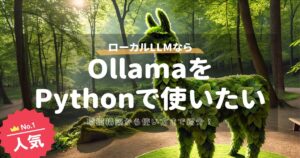
ollama.createを実行するとResponseError: invalid digest formatのエラーが発生しました。
以下のエラーログを確認すると、
SHA256ダイジェストの形式「http://127.0.0.1:11434/api/blobs/sha256:sha256:~」において、
sha256が2回連続で記述されており、無効なフォーマットになっていることがわかります。
DEBUG:httpcore.connection:connect_tcp.started host='127.0.0.1' port=11434 local_address=None timeout=None socket_options=None
DEBUG:httpcore.connection:connect_tcp.complete return_value=<httpcore._backends.sync.SyncStream object at 0x7f07209cace0>
DEBUG:httpcore.http11:send_request_headers.started request=<Request [b'POST']>
DEBUG:httpcore.http11:send_request_headers.complete
DEBUG:httpcore.http11:send_request_body.started request=<Request [b'POST']>
DEBUG:httpcore.http11:send_request_body.failed exception=WriteError(BrokenPipeError(32, 'Broken pipe'))
DEBUG:httpcore.http11:receive_response_headers.started request=<Request [b'POST']>
DEBUG:httpcore.http11:receive_response_headers.complete return_value=(b'HTTP/1.1', 400, b'Bad Request', [(b'Content-Type', b'application/json; charset=utf-8'), (b'Date', b'Sun, 15 Dec 2024 04:50:29 GMT'), (b'Content-Length', b'33'), (b'Connection', b'close')])
INFO:httpx:HTTP Request: POST http://127.0.0.1:11434/api/blobs/sha256:sha256:ee70757d12a0f93d672c5c47a3d2ce5c91b83a0e1f661d9832dc1278658bb628 "HTTP/1.1 400 Bad Request"
DEBUG:httpcore.http11:receive_response_body.started request=<Request [b'POST']>
DEBUG:httpcore.http11:receive_response_body.complete
DEBUG:httpcore.http11:response_closed.started
DEBUG:httpcore.http11:response_closed.complete
---------------------------------------------------------------------------
ResponseError Traceback (most recent call last)
Cell In[4], line 18
1 import ollama
3 modelfile='''
4 FROM ./tokyotech-llm-Llama-3.1-Swallow-8B-Instruct-v0.1-Q4_K_M.gguf
5 TEMPLATE """{{ if .System }}<|start_header_id|>system<|end_header_id|>
(...)
15 PARAMETER stop "<|reserved_special_token"
16 '''
---> 18 ollama.create(model='swallow3_1', modelfile=modelfile)
File /app/.venv/lib/python3.10/site-packages/ollama/_client.py:514, in Client.create(self, model, path, modelfile, quantize, stream)
512 modelfile = self._parse_modelfile(realpath.read_text(), base=realpath.parent)
513 elif modelfile:
--> 514 modelfile = self._parse_modelfile(modelfile)
515 else:
516 raise RequestError('must provide either path or modelfile')
File /app/.venv/lib/python3.10/site-packages/ollama/_client.py:544, in Client._parse_modelfile(self, modelfile, base)
542 path = path if path.is_absolute() else base / path
543 if path.exists():
--> 544 args = f'@{self._create_blob(path)}\n'
545 print(command, args, end='', file=out)
547 return out.getvalue()
File /app/.venv/lib/python3.10/site-packages/ollama/_client.py:561, in Client._create_blob(self, path)
558 digest = f'sha256:{sha256sum.hexdigest()}'
560 with open(path, 'rb') as r:
--> 561 self._request_raw('POST', f'/api/blobs/sha256:{digest}', content=r)
563 return digest
File /app/.venv/lib/python3.10/site-packages/ollama/_client.py:122, in Client._request_raw(self, *args, **kwargs)
120 r.raise_for_status()
121 except httpx.HTTPStatusError as e:
--> 122 raise ResponseError(e.response.text, e.response.status_code) from None
123 return r
ResponseError: invalid digest format
UbuntuのコマンドラインからDockerコンテナ内にアクセスします。
docker exec -it <コンテナ名またはコンテナID> /bin/bash
Ollamaの「_client.py」ファイルを開きます。
nano .venv/lib/python3.10/site-packages/ollama/_client.py
nanoのエディターで、ファイル内のコードを検索をします。
「Ctrl + W」を押して、「Search:」欄にキーワードの「sha256:」を入力後、「Enter」を押すと対象の行が検索できます。
self._request_raw(‘POST’, f’/api/blobs/sha256:{digest}’, content=r)から、「sha256:」を削除します。
修正前のコード
digest = f'sha256:{sha256sum.hexdigest()}'
with open(path, 'rb') as r:
self._request_raw('POST', f'/api/blobs/sha256:{digest}', content=r)
return digest
修正後のコード
digest = f'sha256:{sha256sum.hexdigest()}'
with open(path, 'rb') as r:
self._request_raw('POST', f'/api/blobs/{digest}', content=r)
return digest
「Ctrl+S」で変更を保存して、「Ctrl+X」でnanoエディターを終了します。
コンテナを再起動して、再度ollama.createを実行します。
コマンド実行後にstatus=’success’の表示がされるとollamaのモデル設定が完了です。
import ollama
modelfile='''
FROM ./tokyotech-llm-Llama-3.1-Swallow-8B-Instruct-v0.1-Q4_K_M.gguf
TEMPLATE """{{ if .System }}<|start_header_id|>system<|end_header_id|>
{{ .System }}<|eot_id|>{{ end }}{{ if .Prompt }}<|start_header_id|>user<|end_header_id|>
{{ .Prompt }}<|eot_id|>{{ end }}<|start_header_id|>assistant<|end_header_id|>
{{ .Response }}<|eot_id|>"""
PARAMETER stop "<|start_header_id|>"
PARAMETER stop "<|end_header_id|>"
PARAMETER stop "<|eot_id|>"
PARAMETER stop "<|reserved_special_token"
'''
ollama.create(model='swallow3_1', modelfile=modelfile)
生成AI・LLMのコストでお困りなら
GPUのスペック不足で生成AIの開発が思うように進まないことはありませんか?
そんなときには、高性能なGPUをリーズナブルな価格で使えるGPUクラウドサービスがおすすめです!
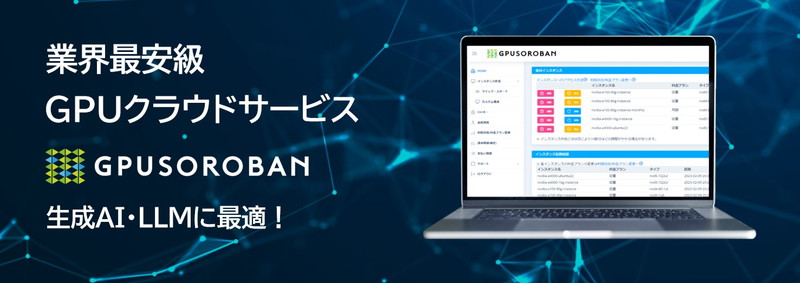
GPUSOROBANは、生成AI・LLM向けの高速GPUを業界最安級の料金で使用することができます。
インターネット環境さえあれば、クラウド環境のGPUサーバーをすぐに利用可能です。
大規模な設備投資の必要がなく、煩雑なサーバー管理からも解放されます。